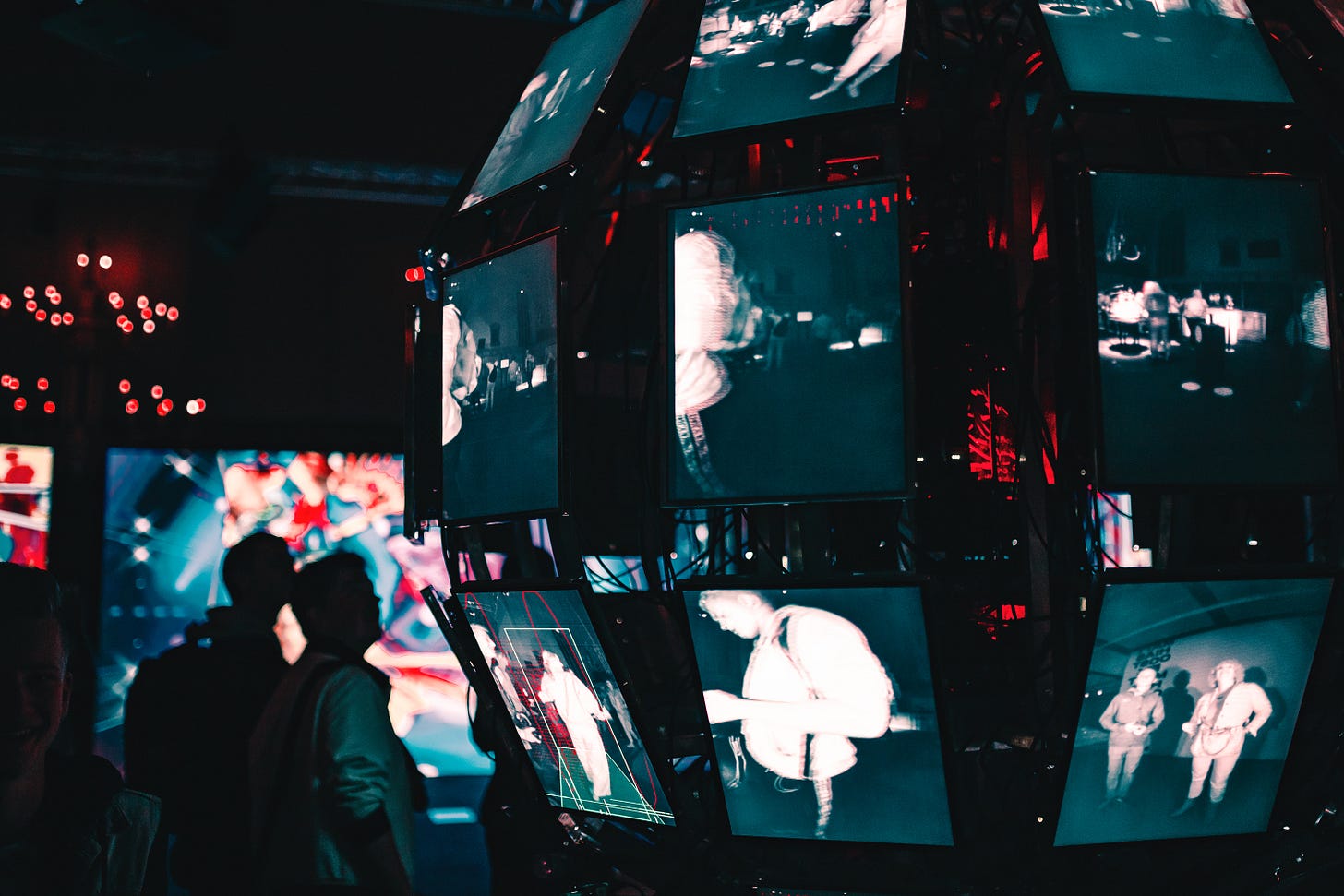
Tis the season for holiday gatherings, mixers and cocktail parties. I am guilty of altering my response to the eternal ice breaker, “So what do you do?”
In my defense, I think it is important to tailor the answer with context for the inquisitive Socrates. For example, if you are in the journalism or writing space I might be a data journalist. You work with data? I am a data analyst. Do I know you from my GIS projects? I am a geospatial analyst. I am not a serial liar, I simply know that it is easy to confuse with abstraction and esoteric blathering.
Occasionally I might find myself in the crosshairs of a gasconader where I am now cornered listening to diatribes on machine learning, artificial intelligence or god forbid being held to task on how someone interpreted something I posted on a social media platform. You know the type. You mention something tangentially linked to virology and suddenly all of the experts masquerading as bankers, teachers, or thespians have an authoritative opinion.
It is the same way about machine learning and AI or probably a host of other topics often spawned in response to my work description.
For starters, we seem to be unaware of our biases. Let’s start there. A brilliant friend of mine, Amy Herman, recently wrote an amazing book, Fixed: How to Perfect the Fine Art of Problem Solving.
With Amy Herman’s Fixed, we now have access to what the FBI, NATO, the State Department, Interpol, Scotland Yard, and many more organizations and their leaders have been using to solve their most intractable problems.
Amy shares a slide similar to this one:
We see these images and regardless of what we may or may not know about history we regard them as settled fact. Two pivotal presidents early in the history of the formation of the United States.
Look at the next image. Politics aside, now what happens? There is an emotion no matter what side of the aisle you reside. It might be positive it might be negative but I guarantee you feel a certain way. You have lived through the presidency of one of these presidents. That feeling? That is what you bring into your thoughts on a myriad of other tangential topics—I have introduced you to your implicit bias.
This is important. Here is why. The data you bring into your analyses or that is beneath the surface of visualizations or graphics you consume—all biased. You make decisions (even if working with raw data) about the databases you access, the datasets you include, the datasets you exclude, what level of granularity, how you formulate your data question and on and on.
A recent twitter comment I posted was a perfect example.
Racial inequity. What if we are answering the wrong questions?
I thought of this question as a little example of hindsight bias. It is easier to see our way forward when we assume we knew something all along. Well of course we are asking and answering the wrong questions. We know this to be true. But do we?
If that is the case, why do we still slice our data by race? Is it a self fulfilling prophecy? Stay with me for a minute. Counterfactual thinking considers alternate possibilities—whereas counterfactual causal models define treatment effects of race by manipulating identical units such as a person, neighborhood, school.
“We think of a cause as something that makes a difference, and the difference it makes must be a difference from what would have happened without it. Had it been absent, its effects – some of them, at least, and usually all – would have been absent as well”—definition of counterfactual analysis, David Lewis
This manipulation requires a biologic or genetic concept of race that is nonsensical and does not exist. Fans of Saturday Night Live (of a certain age) might recall Eddie Murphy’s skit, “White Like Me,”where he examined what his life would be like as a white man. Issa Kohler-Hausmann steps us through the fallacies of applying counterfactual causal models to issues of race.
Eddie Murphy’s race is an “immutable characteristic” unable to be manipulated as a unit of race, but examined as a “trait of the unit of interest”.
It [counterfactual model] is wrong because to fit the rigor of the counterfactual model of a clearly defined treatment on otherwise identical units, we must reduce race to only the signs of the category, meaning we must think race is skin color, or phenotype, or other ways we identify group status. And that is a concept mistake if one subscribes to a constructivist, as opposed to a biological or genetic, conception of race.
The counterfactual causal model of discrimination is based on a flawed theory of what the category of race references, how it produces effects in the world, and what is meant when we say it is wrong to make decisions of import because of race. I argue that DISCRIMINATION is a thick ethical concept that at once describes and evaluates the actions to which it is applied, and therefore, we cannot detect actions as discriminatory by identifying a relation of counterfactual causality; we can do so only by reasoning about the action’s distinctive wrongfulness by referencing what constitutes the very categories that are the objects of concern.—Issa Kohler-Hausmann, Eddie Murphy and the Dangers of Counterfactual Causal Thinking about Detecting Racial Discrimination
I ascribe to a constructivist theory of race and because it is too complex to respond in a tweet length comment, it is almost always misinterpreted.
A constructivist rejects the notion that racial categories in the United States are constituted by genetic or biological facts, and instead holds that what now seem like obvious, taken-for-granted categories of racial difference were constructed over hundreds of years of historical practices starting with chattel slavery and colonization. Categories such as “whiteness” and “blackness” were forged through social relations such as forced labor, colonization, immigration, and Jim Crow; they were contested and policed through many institutions including immigration laws, housing and education segregation, violent regulation of social and intimate relations, and hoarding of occupational and economic opportunity.
I don’t discredit colleagues that have been elevated to speak on such matters. Large predominantly white companies, academic centers, and institutions are working in earnest to occupy the space of trying to right centuries of being on the wrong side of history with well meaning discussion panels or “playbooks”. I don’t discredit anyone because it is the collective that hoisted them up and invited them to the microphone.
I don’t need anyone to educate me on the plight of marginalized people and how it is their voices we need to be listening to and elevating. I mean it is easy for these voices to grab attention right? Nope. I listen as do others, because we can see the bias built into creating deep buckets of people based on poverty and other low-hanging social variables—and then highlighting race. We are unable to create policies that will help without asking the hard questions of how our own government created the social policies now being challenged and dismantled.
If we accept that race is such a salient vector of social life that it is incoherent to conceptualize the causal effect of race by asking a person to be the exact same person but for race at a particular moment in time, then it is similarly incoherent to conceptualize the causal effect of race by imagining decision-makers to perceive two candidates as otherwise identical but for race for similar reasons. The catch in the thought experiment is not biology making race “immutable,” but history, economics, and sociology making race a fundamental structuring category of thought, perception, action, and experience in the United States.
I am a Diversity Committee Chair at SciPy 2022. I think careful questions, analysis and story can help us make inroads and narrow the gap of what we are all attempting to achieve collectively.
Initially, the hindsight bias and counterfactual thinking would seem to be inconsistent with each other. If counterfactuals represent awareness of alternative possibilities, shouldn’t they actually reduce the hindsight bias?—Adam D. Galinsky et al. Finding meaning from mutability.
Readings from the work on counterfactual thinking, intersectionality, and analyses of US Census Data have sparked an important narrative.
If we consider the counterfactuals also provide casual explanations, and causal explanations increase a subjective state of certainty, then counterfactual thinking and the hindsight bias become compatible with each other—Adam D. Galinsky et al. Finding meaning from mutability.
I am speaking about Engaging Story with Place on 12/10/21 at 1:00 PM.
Discussions of inequity have a history and a place--how can we bring story and place together with data and storytelling?
The recording will be available for download after the date. There is a cost—$50—but if you are subscriber, reach out to me and I will give you an access code for either the live event or the recording. I have copyrighted works and proprietary data in this discussion and think that it is only fair that I restrict viewing.
It is with the lens of racial inquiry and inequity that I share with you the:
Artificial Intelligence Incident Database
Scientists Built an AI to Give Ethical Advice, But It Turned Out Super Racist
“We’ve all been in situations where we had to make tough ethical decisions. Why not dodge that pesky responsibility by outsourcing the choice to a machine learning algorithm?”
Word counts in incident reports ranked by their totals:
I like to share at least one interesting database or resource in the newsletter each issue. Let me know what you think…
*As an Amazon associate, I may receive a small payment for links to books included in the text.